Step into the future of content creation, where the transformative power of AI-generated content is reshaping industries and pushing the boundaries of creativity.
From news articles to product descriptions, AI algorithms are producing vast amounts of text that often mimic human writing styles.
However, with this surge in machine-generated content, the need for reliable AI content detectors has become increasingly crucial.
These detectors play a vital role in distinguishing between human and AI-generated text, ensuring the integrity and authenticity of the content we consume.
Also Read: Fix IP Address issues
In this article, we will delve into the significance of the AI content detectorand explore how they work to identify machine-generated text.
So, let's dive in and uncover the secrets behind these powerful AI tools.
Understanding AI Content Detection Technology
To grasp the concept of AI content detectors, let's dive into what happens behind the scenes. These detectors primarily rely on analyzing the context preceding a specific word to make predictions about AI-generated content.
Consider the sentence,
"After a long day at work, I love to relax with a good book and a warm cup of ___."
Drawing from extensive training on a diverse dataset of 117 million data points, the AI model, such as the GPT-3 language model, has developed a keen ability to identify patterns and connections within word sequences.
In this particular example, the word "tea" emerges as the most frequently predicted option, with a 41% chance based on the training data.
The AI model refers back to its training data and examines the patterns in the word set's context. It recognizes that words like "tea" and "coffee" are often followed by the word "cup of."
By calculating the probability of each word being the next predicted word based on these patterns, the AI model makes its prediction.
So, How do AI Content Detectors Work?
In terms of how AI content detectors function, the process is relatively straightforward.
Let’s walk through an example to illustrate this process.
1. We will start by generating an article or any piece of text using an AI text generator. Open up a GPT Writer and generate an article, blog post, or any piece of content.
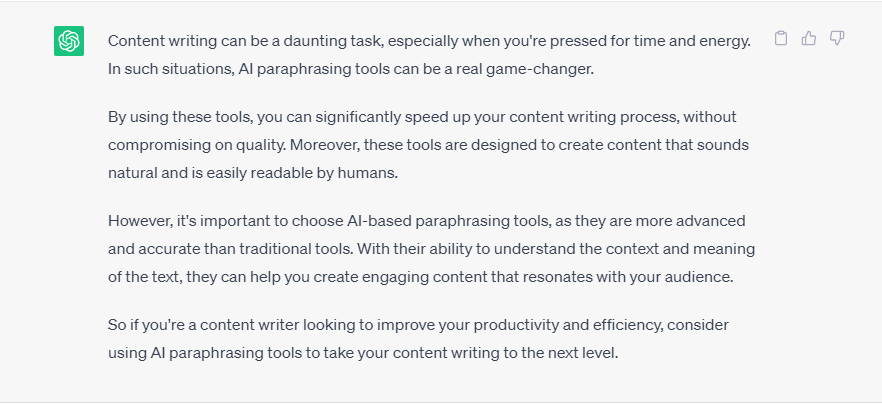
2. Once we have the generated text, we can proceed to check its originality using AI content detectors.
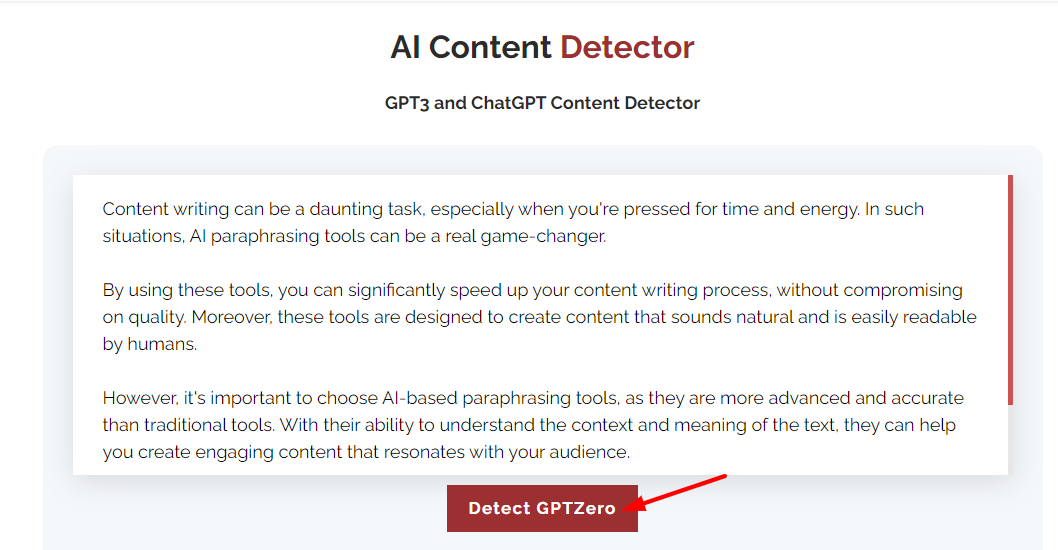
3. The AI content detectors begin by analyzing the text sentence by sentence. They calculate predictions based on patterns and word context.
4. If a significant portion consistently selects the most predictable words or phrases, it raises suspicion that the text might be artificially generated.
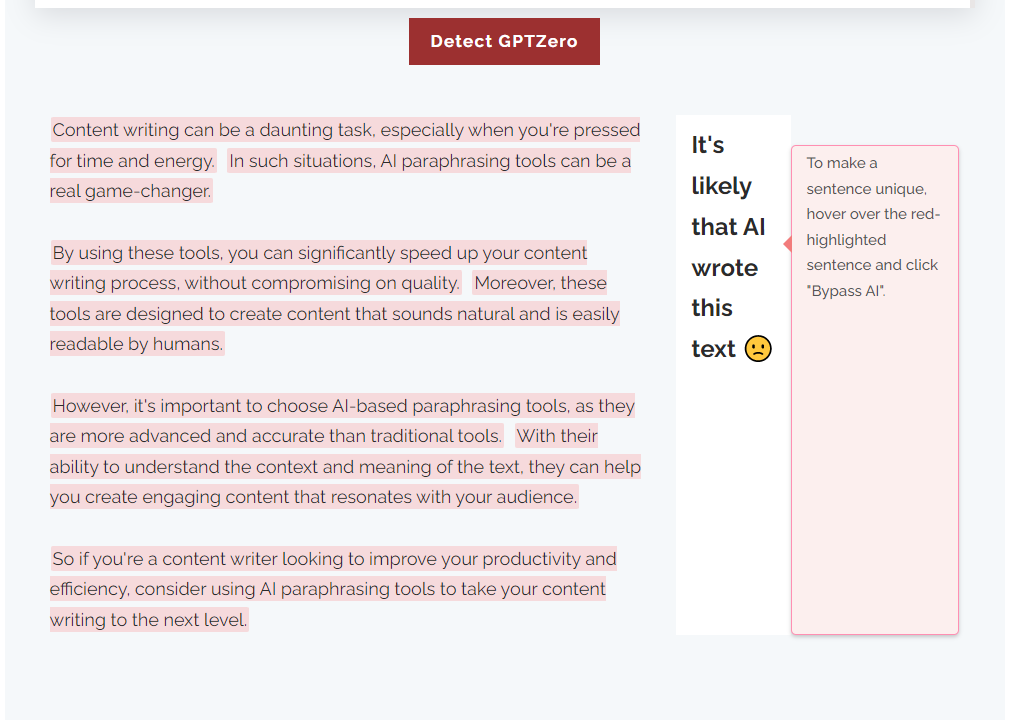
See how this content detector tool has highlighted the GPT written content in a matter of seconds.
In response to AI content detection, you can make use of the AI content bypass tool to rectify this issue.
This AI tool utilizes advanced techniques to generate content that can bypass the detection algorithms used by AI content detectors.
To make AI-written sentences unique, simply hover over the red-highlighted sentences one after another and click on "Bypass AI".
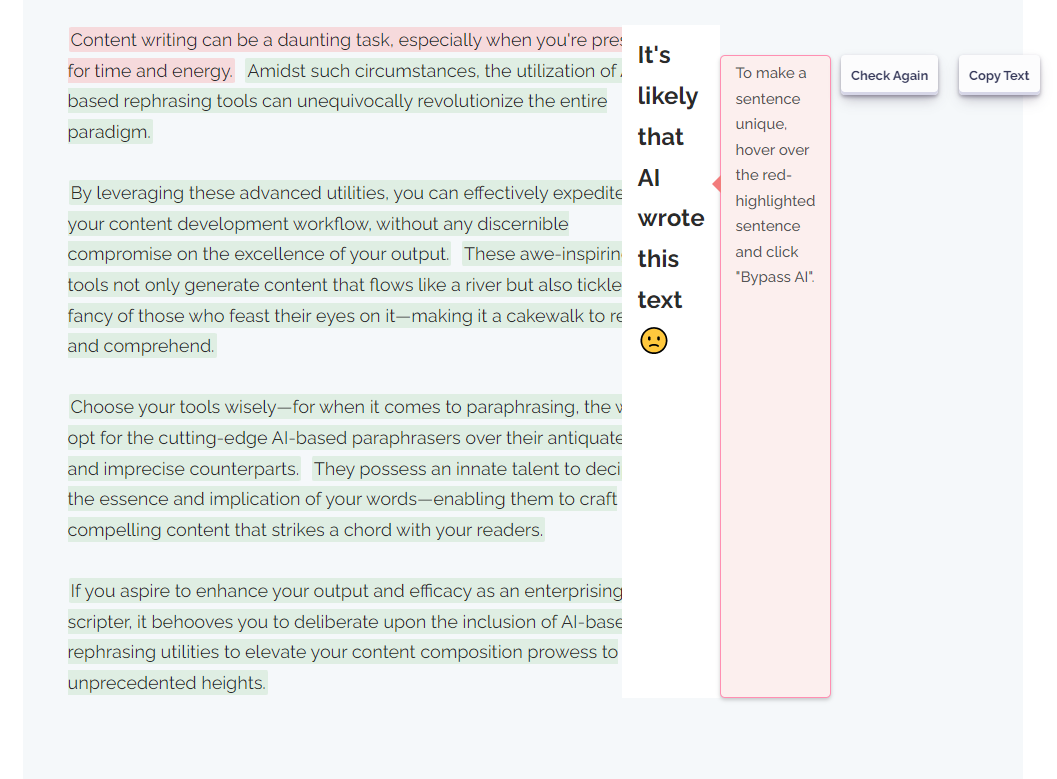
Now you can check the content again for AI detection to make sure it is now unique and original. Simply click on “check again” and see the results:
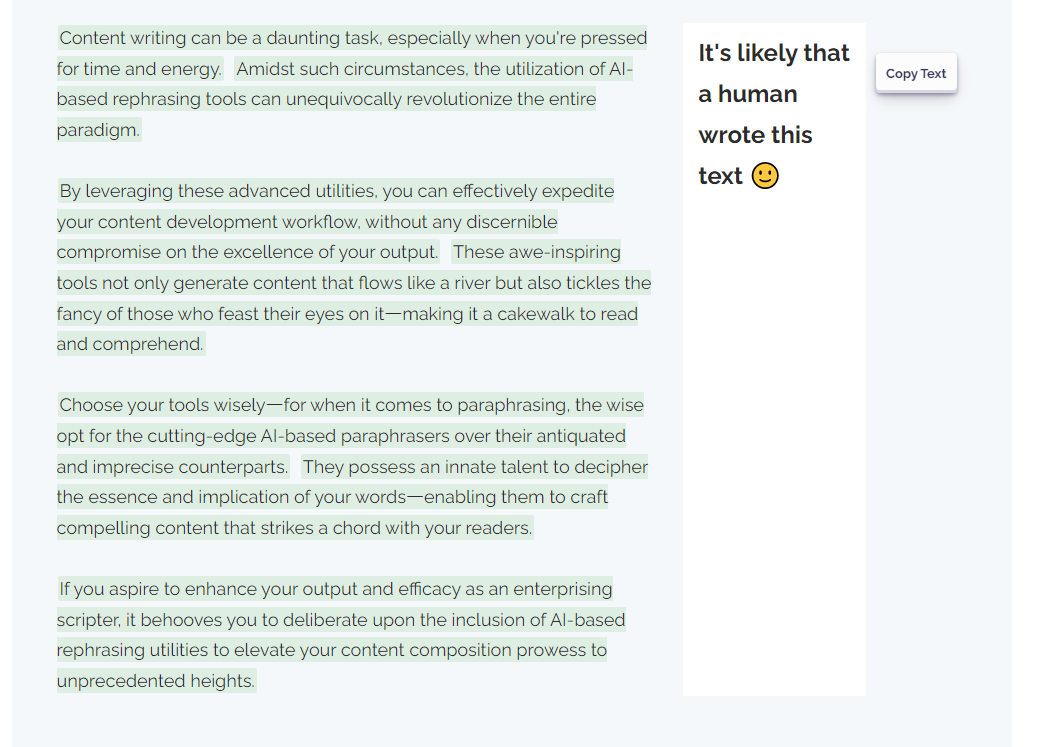
Side-note
It's important to note that authentic human writing tends to exhibit complexity and employs unpredictable and creative language. This natural variation makes it distinct from machine-generated content.
Key challenges in accurate content detection
Despite the advancements in AI content detection, there are still challenges to overcome.
The evolving complexity of language models means that AI's predictive capabilities make it harder to discern between human and machine-generated text.
As the volume of training data increases, the variability in AI-generated content also expands, complicating the detection process.
Analyzing large chunks of text according to the predictive regeneration capability of AI models can provide insights.
If a sampled text consistently relies on the most predictable words throughout paragraphs, it is likely machine-generated.
In contrast, skilled human writers often employ complex language and provide unpredictable explanations, setting their work apart from artificial writing.
While the current methodology for detecting AI-generated content based on word context is relatively simple, the ongoing advancements in AI technologycall for continued research and adaptation to effectively identify machine-generated text.
By understanding the concept, inner workings, and challenges of AI content detection, we gain valuable insights into the fascinating world of automated content generation.
Limitations and Flaws of AI Detection: Real-life Examples
Despite significant advancements, AI detection tools are not immune to flaws and can yield inaccurate results and false positives.
Consider a student who was wrongly accused of cheating on his history exam due to an unreliable AI content detector.
This unfortunate incident sheds light on the limitations and potential harm associated with relying solely on these technologies for distinguishing human-generated content from AI-generated content.
Let's explore some other common scenarios where AI detection falls short:
1. Social media moderation
Prominent platforms like Facebook and Twitter employ AI-powered software to identify and flag inappropriate or harmful content. However, these systems often mistakenly categorize innocent posts as violations while allowing malicious content to go undetected, undermining their effectiveness.
2. Educational institutions
Many schools rely on plagiarism checkers that utilize machine learning algorithms to identify instances of copied work. Unfortunately, these systems occasionally misidentify original writing as plagiarized material, resulting in unfounded accusations against students and potential damage to their academic reputation.
3. Hiring processes
Companies increasingly integrate natural language processing (NLP) tools into their recruitment procedures. However, if these tools fail to recognize specific dialects or cultural nuances within submitted documents, they can inadvertently discriminate against certain applicants. This highlights the importance of ensuring that AI detectors are sensitive to diverse linguistic and cultural variations.
These real-life examples demonstrate the flaws and limitations that exist within AI detection systems.
While these technologies offer valuable assistance, it is essential to acknowledge their shortcomings and work towards improving their accuracy and reliability.
Striking a balance between AI assistance and human judgment can help mitigate the risks and ensure fair outcomes in various domains where AI detection is utilized.
Reasons Behind AI Content Detector's Limitations
In a fascinating twist, AI content detection tools utilize AI to identify AI-generated content. Why do these seemingly advanced AI content detection tools sometimes fall short of expectations? Let's find out.
1. Insufficient Training Data
AI content detectors heavily rely on comprehensive and diverse training data. However, one of the main limitations they encounter is the scarcity of quality training data. Without an ample and varied dataset, these systems may struggle to accurately identify and differentiate between various types of content.
2. Boundaries of Language Models
While these models have made significant strides in natural language processing and understanding, they still struggle with contextual nuances, sarcasm, irony, and cultural references. As a result, they might fail to grasp the full meaning or intent behind certain phrases or sentences, potentially leading to misclassifications.
3. Ever-Advancing Writing Techniques
The dynamic nature of AI-driven content creation poses a constant demand for updates and refinements in detection algorithms. Without regular adjustments, content detectors risk falling behind and becoming less effective in identifying and flagging problematic content.
Navigating the Future of AI Content Detection
While AI content detectors have proven to be valuable tools in combating misinformation and maintaining online integrity, it is crucial to acknowledge and address their limitations.
Enhancing training data diversity, refining language models to better understand nuanced language, and establishing agile update mechanisms are essential steps toward improving the effectiveness of AI content detectors.
As AI content continues to rise, it becomes imperative to evolve AI detection techniques to ensure a safer and more reliable digital landscape.